Comparing Multispectral and Hyperspectral Imagery in GIS
In the world of geographic information systems (GIS), using remote sensing is key. Two main imaging types, multispectral and hyperspectral, are getting a lot of attention. They help us understand things like how crops grow and how cities are planned. This article will look at what makes these imaging types different, their benefits, and their challenges. It’s to help GIS experts pick the right tool for their job.
Key Takeaways
- Multispectral and hyperspectral imagery are two distinct remote sensing technologies used in GIS applications.
- Multispectral imagery captures data in a few broad spectral bands, while hyperspectral imagery captures detailed spectral information in numerous narrow bands.
- Understanding the unique characteristics and applications of these imaging techniques is essential for GIS professionals to choose the most suitable solution for their needs.
- Multispectral imagery excels in simplicity, cost-effectiveness, and faster processing, while hyperspectral imagery offers enhanced material detection and improved classification accuracy.
- Careful consideration of factors such as spectral resolution, spatial resolution, and temporal resolution is crucial when selecting the appropriate imaging technology for GIS applications.
Introduction to Multispectral and Hyperspectral Imagery
In the world of geographic information systems (GIS), advanced imaging is key. Multispectral and hyperspectral imagery are at the forefront. They capture data across the electromagnetic spectrum for many uses.
Definition of Multispectral Imagery
Multispectral imagery uses remote sensing to capture data in specific spectral bands. These bands range from a few to dozens. This lets us analyze different features and materials on Earth.
Definition of Hyperspectral Imagery
Hyperspectral imagery is more advanced. It captures hundreds or thousands of spectral bands. This high-resolution data gives us a detailed look at what we’re observing.
Importance of Imagery in GIS
Multispectral and hyperspectral imagery are vital in GIS. They help us map, monitor, and analyze various environmental, agricultural, and urban features. These tools are essential for land-use planning, resource management, and environmental monitoring.
“Multispectral and hyperspectral imagery provide a wealth of information that can significantly enhance our understanding of the world around us.”
GIS professionals use these technologies to make better decisions. They improve resource use and support sustainable development.
Key Differences Between Multispectral and Hyperspectral Imagery
In the world of geographic information systems (GIS), choosing between multispectral and hyperspectral imagery matters a lot. These two remote sensing technologies differ in image resolution, spectral bands, and data collection frequency.
Spectral Resolution
Multispectral sensors capture data in a few broad spectral bands, usually 3 to 10. Hyperspectral sensors, however, record data across hundreds of narrow spectral bands. This gives hyperspectral a more detailed and comprehensive view of the electromagnetic spectrum.
Spatial Resolution
Multispectral sensors have a higher spatial resolution. They can capture more detailed and precise information about an area. Hyperspectral sensors, though, sacrifice some spatial resolution for their enhanced spectral capabilities.
Temporal Resolution
The frequency of data collection, or temporal resolution, also differs. Multispectral sensors collect data more frequently, allowing for regular monitoring. Hyperspectral sensors, due to their complex data processing, have a lower temporal resolution.
Characteristic | Multispectral Imagery | Hyperspectral Imagery |
---|---|---|
Spectral Resolution | Broad spectral bands (3-10) | Narrow spectral bands (hundreds) |
Spatial Resolution | Higher spatial resolution | Lower spatial resolution |
Temporal Resolution | Higher data collection frequency | Lower data collection frequency |
Knowing the differences between multispectral and hyperspectral imagery is key. It helps choose the right remote sensing technology for GIS applications. This ensures the data collected meets the project’s needs.
Applications of Multispectral Imagery
Multispectral imagery is a powerful tool used in many fields. It helps in agriculture, land use mapping, and forestry management. It captures data in different wavelength bands, giving insights for important decisions.
Agriculture Monitoring
In agriculture, multispectral imagery is key for crop health checks. It uses vegetation indices to monitor crops. Farmers can spot early stress signs and improve yields.
This tech also helps find weeds, pests, and diseases. It makes crop management more efficient and sustainable.
Land Cover Classification
Multispectral imagery is vital for land use mapping. It helps classify different land types like cities, forests, and farms. It’s key for urban planning, environmental tracking, and resource use.
Forestry Management
In forestry, multispectral imagery is a key tool. It monitors vegetation and forest health. It lets foresters spot issues, track deforestation, and plan for forest care.
Multispectral imagery is a must-have for GIS experts. It helps solve many problems in agriculture, land use, and forestry.
Applications of Hyperspectral Imagery
Hyperspectral imagery is used in many fields, not just in agriculture and forestry. It helps in geological mapping, environmental monitoring, and urban planning. This technology changes how we see and interact with our world.
Mineral Exploration
Hyperspectral sensors find tiny changes in the Earth’s surface. They are key for finding minerals like gold and copper. This helps geologists map the Earth more accurately and safely.
Environmental Monitoring
Hyperspectral imagery fights against pollution. It can spot pollutants and check water and plant health. This helps scientists track oil spills and greenhouse gases, leading to better environmental care.
Urban Planning
The urban heat island effect makes cities hotter than the countryside. Hyperspectral sensors map these temperature differences. This helps planners design cooler, greener cities for better living.
Hyperspectral imaging will keep improving. We’ll see new uses in mapping, pollution detection, and city planning. It will change how we interact with our environment.
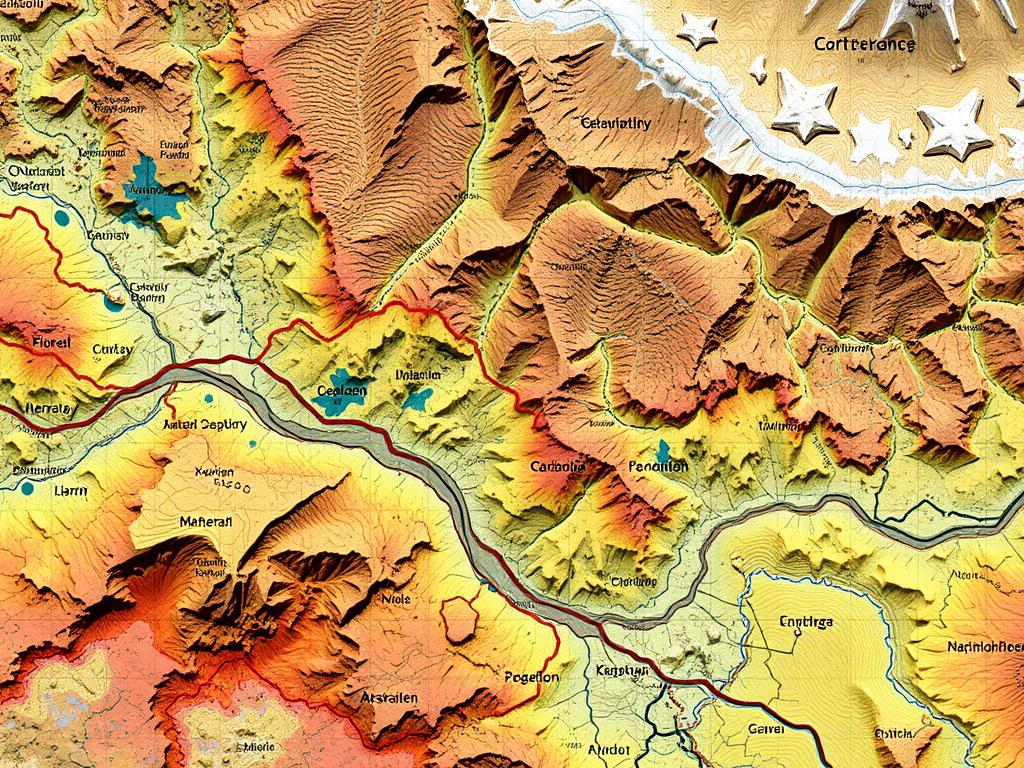
Advantages of Multispectral Imagery
Multispectral sensors have big advantages over hyperspectral imagery. They are simpler and cheaper to use. This makes them great for many tasks. They are known for being cost-effective remote sensing, and multispectral sensors.
Simplicity and Cost-Effectiveness
Multispectral imagery is easy to work with. It has fewer bands than hyperspectral, so it’s simpler to process. This means it’s cheaper and easier to use, reaching more people and groups.
Faster Processing Time
Multispectral data is easier to handle, so it’s processed faster. This is a big plus for urgent tasks. It helps in big projects where speed is key.
Lower Data Volume
Multispectral sensors make less data than hyperspectral ones. This makes it easier to store and send. It’s perfect for projects with limited resources.
Multispectral imagery is a top choice for many uses. It’s great for cost-effective remote sensing and big projects. It’s all about data processing efficiency and using multispectral sensors.
Advantages of Hyperspectral Imagery
Hyperspectral imagery has many benefits over traditional methods. It’s great for various GIS uses. Key benefits include better material detection, detailed spectral analysis, and higher accuracy in classification.
Enhanced Material Detection
Hyperspectral sensors can spot small differences in materials. This is something multispectral systems can’t do. It helps map materials like minerals, plants, and buildings more accurately.
By looking at the spectral signatures of these materials, hyperspectral imagery offers high-precision mapping. This detailed view of the landscape is very useful.
Detailed Spectral Analysis
Hyperspectral systems collect data in hundreds of narrow bands. This gives a deep look into the properties of materials. It’s better than multispectral systems that only capture a few broad bands.
This detailed spectral data helps identify and classify materials more accurately. It’s a big help for researchers and analysts to understand the environment better.
Improved Classification Accuracy
Hyperspectral imagery’s ability to detect and analyze materials leads to better classification. This is especially true in complex settings. It’s vital for tasks like precision farming, environmental monitoring, and urban planning.
Having accurate land cover and use information is key. It helps make better decisions and manage resources more effectively.
Advantage | Description |
---|---|
Enhanced Material Detection | Hyperspectral sensors can capture subtle differences in material identification, enabling more precise high-precision mapping and characterization of surface materials using their unique spectral signatures. |
Detailed Spectral Analysis | Hyperspectral imagery provides a wealth of spectral data across hundreds of narrow, contiguous bands, allowing for in-depth analysis of the physical and chemical properties of materials. |
Improved Classification Accuracy | The enhanced material detection and detailed spectral analysis of hyperspectral imagery lead to significantly improved classification accuracy, particularly in complex environments. |
Hyperspectral imagery is a valuable tool for GIS applications. It’s great for material identification, spectral signatures analysis, and high-precision mapping. It also improves classification accuracy. As the technology gets better, its uses in GIS will grow even more.
Challenges of Multispectral Imagery
Multispectral imagery has many benefits but also faces challenges. One big issue is the spectral limitations it has.
Limited Spectral Bands
Multispectral sensors capture data in a few spectral bands, usually 3 to 10. This limited spectral resolution makes it hard to tell apart some materials or land types. This is because they might reflect light in similar ways in the available bands.
Less Detailed Data
The data resolution of multispectral imagery is not as high as hyperspectral imagery. Hyperspectral imagery gives a detailed spectral profile. This lower detail makes it tough to solve some application-specific challenges, like precise mineral identification or detailed urban land-use mapping.
Application Constraints
Because of the spectral resolution and data resolution limits, multispectral imagery isn’t always the best choice. For applications needing a deeper understanding of spectral properties, hyperspectral imagery might be better. Even though it has its own challenges.
To overcome these issues, researchers and developers are always improving multispectral sensors. They’re looking to add more spectral bands and boost the data resolution. They aim to do this without making the systems too complex or expensive.
Challenges of Hyperspectral Imagery
Hyperspectral imagery gives us deep insights but comes with big challenges. The main hurdles are dealing with lots of data, complex processing, and higher costs for getting and analyzing it.
High Data Volume
Hyperspectral sensors gather detailed spectral info across hundreds of narrow bands. This creates huge data sets. Handling and storing this data is a big challenge for GIS experts. They need advanced algorithms to make sense of it all.
Complex Processing Requirements
Getting useful info from hyperspectral data needs complex advanced processing algorithms. These algorithms require a lot of computing power. This makes processing hyperspectral imagery slow and resource-heavy.
Higher Costs
The cost of getting and keeping hyperspectral sensor costs is much higher than traditional sensors. This is because of the advanced tech and special hardware needed. These costs can stop some groups from using hyperspectral imagery in GIS.
Even with these challenges, hyperspectral data’s rich info keeps pushing GIS tech forward. As the field grows, the costs and complexity of hyperspectral imagery should drop. This will make it more available and useful for GIS projects.
Case Studies Showcasing Multispectral Imagery
Multispectral imagery is a game-changer in precision agriculture and ecosystem analysis. Let’s dive into two case studies that show its impact.
Precision Agriculture Case Study
A leading agricultural research institute used multispectral imagery to boost crop yields. They analyzed spectral signatures to spot nutrient deficiencies and pests early. This led to better farming practices and higher yields.
By using multispectral imagery, farmers could use resources like water and fertilizers more wisely. This made farming more productive and reduced environmental harm. It’s a win for farmers and the planet.
Ecosystem Analysis Case Study
Multispectral imagery is key in habitat monitoring and ecosystem analysis. A renowned conservation group used it to track vegetation changes and wetland health. They also detected deforestation early.
By studying plant species’ spectral signatures, they mapped ecosystems accurately. This helped guide conservation efforts and land management. It’s a powerful tool for protecting our environment.
These case studies highlight multispectral applications‘ power in solving real-world problems. As technology advances, we’ll see even more breakthroughs in various fields.
Case Studies Showcasing Hyperspectral Imagery
Hyperspectral technology offers advanced capabilities beyond multispectral imagery. It’s especially useful for tackling big environmental issues. For example, it’s great for forest cover change detection and aquatic ecosystem health assessment.
Deforestation Monitoring
Hyperspectral sensors can spot the unique spectral signs of plants. This helps map forest areas accurately. It’s a key tool for tracking forest cover change detection.
It alerts us to deforestation early. This lets us focus conservation efforts where they’re needed most. By spotting forest degradation early, we can act fast to save these important areas.
Water Quality Assessment
Hyperspectral applications also excel in keeping water ecosystems healthy. It gives us detailed info on water quality, like chlorophyll levels and turbidity. This is vital for aquatic ecosystem health monitoring.
It helps water managers spot pollution issues early. With hyperspectral data, we can understand the complex water environments better. This knowledge is key to protecting our water resources.
These examples show how hyperspectral imagery can change the game for the environment. As it keeps improving, we’ll see more amazing uses in forest and water management.
Future Trends in Imaging Technology
The world of geospatial information systems (GIS) is changing fast. New technologies in imaging are making big waves. We’re seeing better sensors and more use of artificial intelligence (AI). This is great news for GIS experts and researchers.
Advances in Sensor Technology
New sensors are coming that will change how we collect and use spatial data. They will be able to see more details and map the Earth more accurately. Plus, they’ll be easier to carry and use, making data collection cheaper and more accessible.
Integration with Machine Learning
AI and remote sensing are joining forces, changing how we understand geospatial data. Machine learning will help us classify and analyze images faster and better. This will lead to new uses in environmental monitoring, urban planning, and precision agriculture.
Expansion in Application Areas
- New imaging tech will open up many new GIS uses. Next-generation sensors and AI will bring new chances in:
- Disaster management and response
- Infrastructure planning and asset monitoring
- Biodiversity conservation and ecosystem management
- As AI in remote sensing gets better, we’ll see even more emerging GIS applications. This will change how we see and interact with our world.
The future of GIS imaging is looking bright and full of possibilities. By using these new tools, GIS experts can do their jobs better. This will help drive progress in many fields and industries.
Conclusion: Choosing the Right Imagery for GIS Applications
When picking between multispectral and hyperspectral imagery, think about what your GIS project needs. You should look at the level of detail needed, how complex the analysis is, and what resources you have.
Considerations for Decision Making
For your GIS project, decide if you need a lot of detail. Multispectral imagery is good for things like watching crops or mapping land use. It’s cheaper and quicker. But, for detailed work like finding minerals or tracking the environment, hyperspectral imagery is better.
Summary of Key Points
We’ve talked about the good and bad of multispectral and hyperspectral imagery in GIS. Multispectral is simple, cheap, and fast, great for many uses. Hyperspectral gives more detail and accuracy but is more complex and expensive.
Future Implications for GIS Usage
GIS technology is getting better, and so is the use of advanced images like multispectral and hyperspectral. These will be key in fields like farming, city planning, and watching the environment. With new tech and learning tools, GIS will help us make better decisions faster and more accurately.